Dynamic medical imaging in 4D typically requires motion estimation of the organs. With respect to the spatial-temporal information of the 3D volume sampled over multiple time points, one may assess structural and functional property of the target organ. Our early study in CVPR 2020 shows that, with limited number of temporally sampled phase images, one can reconstruct the organ motion trajectory of high resolution both spatially and temporally, via interpolation in a well-encoded latent space.
Many motion estimation methods are image based, which optimize the motion field by evaluating in the entire image space directly and are thus prone to implausible outputs especially in the presence of large motion. An alternative solution based on sparse landmarks can alleviate this concern. Specifically, we propose a two-stage motion estimation framework of Dense-Sparse-Dense (DSD). First, we extract sparse landmarks from the dense image to represent the target organ. Second, we construct the dense motion field, given the extracted sparse landmarks and their corresponding displacements across different time points.
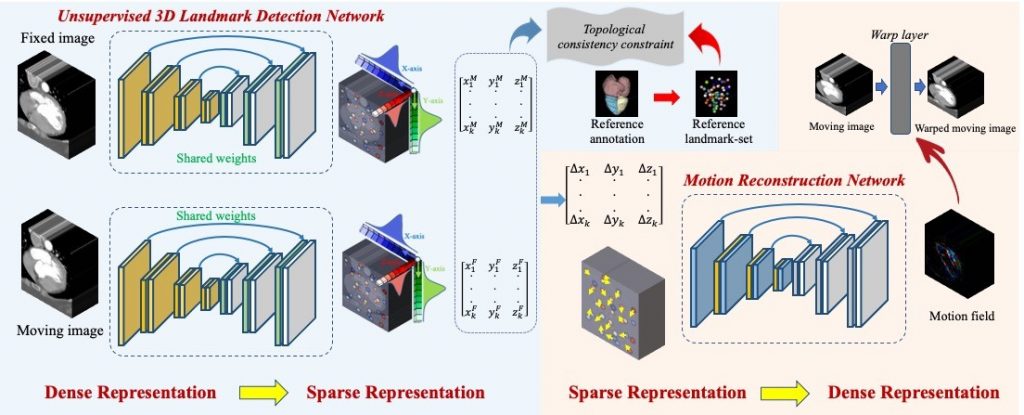
The bottleneck for DSD relates to landmark detection. It is non-trivial for human raters to label corresponding landmarks in different phase images, which is typically required to serve as ground-truth in supervised learning. To tackle this issue, we design an unsupervised 3D landmark detection network. The detector is pre-trained with self-supervised representation learning, to focus its attention toward the motion-affected organ. Several losses are carefully designed to ensure that the landmarks are spatially sparse yet anatomically informative for the target organ. Our experimental results demonstrate that the DSD solution can complete landmark detection and then motion estimation without any need of manual annotation.
For more details of this work, please refer to Guo et al.