One of the most popular trends in medical image analysis is to apply deep learning techniques to computer-aided diagnosis (CAD). However, it is evident now that a large number of manually labeled data is often a must to train a properly functioning deep network. This demand for supervision data and labels is a major bottleneck, since collecting a large number of annotations from experienced experts can be time-consuming and expensive.
In this work, we aim to demonstrate that the eye movement of radiologists when reading medical images can be a new form of supervision to train the deep-learning-based CAD system. Particularly, we record the tracks of the radiologists’ gaze when they are reading images. The gaze information is processed and then used to supervise the deep network’s attention via an attention consistency module.
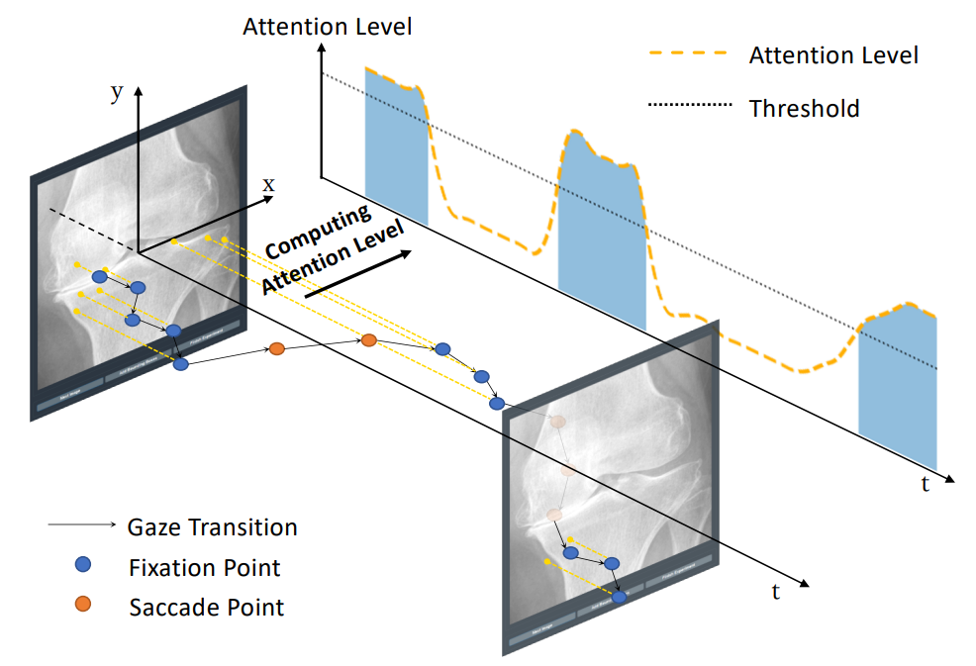
We are among the first to use radiologist’s gaze to build deep-learning-based CAD systems. While tracking eye movement is not completely new to the field of radiology, we have successfully conducted this pilot study and achieved superior performance in the CAD task of radiograph assessment for osteoarthritis.
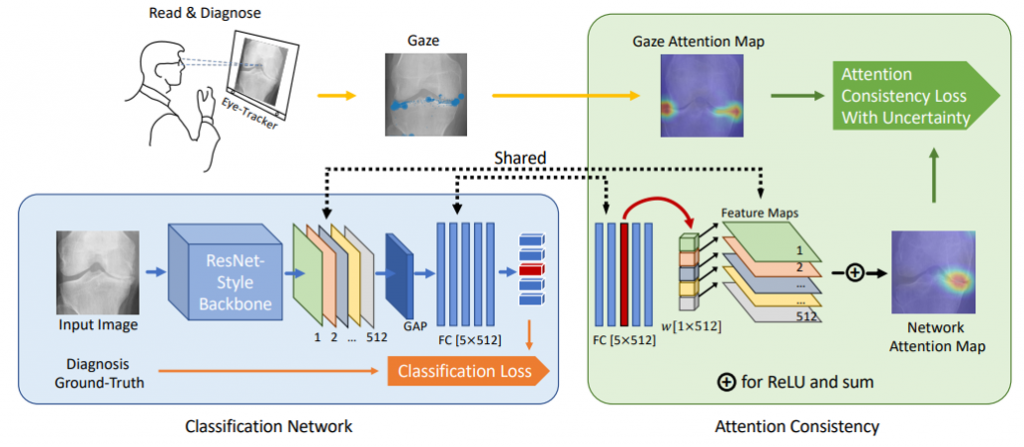
The setup in our lab allows the radiologists to read images without being distorted or interrupted by the gaze tracker. Therefore, our solution can work as a general-purpose plug-in to current clinical workflows and numerous applications. Meanwhile, with our released toolkit, many other labs can initiate their gaze-based researches easily and efficiently.
For more details of this work, please refer to Wang et al.