Knee osteoarthritis (OA) is the most common osteoarthritis and a leading cause of disability. Cartilage defects are regarded as major manifestations of knee OA. However, the cartilage is a thin curved layer, implying that only a small portion of voxels in knee MRI can contribute to the cartilage defect assessment.
To tackle the computation challenges, we model the cartilages structure and appearance from knee MRI into a unified graph representation, which is capable of handling highly diverse clinical data. We also design a non-Euclidean cartilage surface network (CSNet) powered by the self-attention mechanism to derive the cartilage assessment result accurately and robustly.
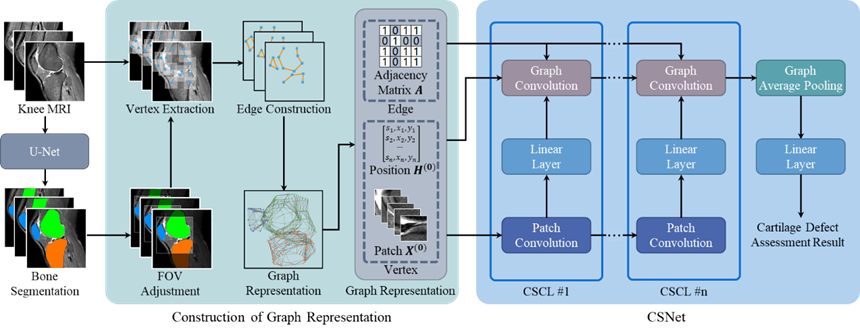
In this way, we effectively combine the cartilage shapes and appearance features for knee cartilage defect assessment at the subject, slice and patch levels. We have collaborated with clincians to demonstrate that our method delivers superior performance in handling heterogeneous knee MRI data acquired in clinical routines.
For more details of this work, please refer to Zhuang et al.